Ise AI Insights #02Aligning Gen AI Tools to Business Metrics
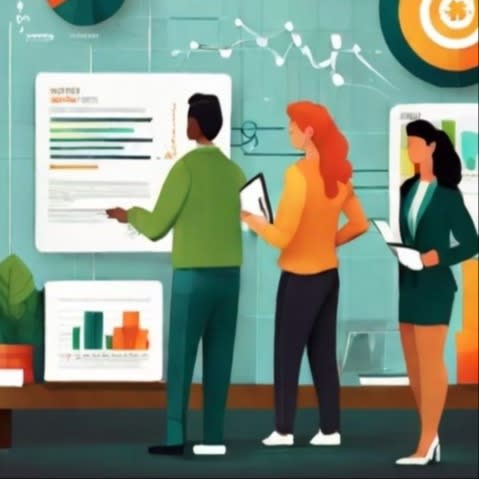
(Ise is pronounced “ee-say”)
In our last article, we discussed why enterprise users may hesitate to widely adopt Generative AI (“Gen AI”) and introduced the concept of an AI Intern. You can revisit the framework using the link here. In this article, we will dissect the first of three Gen AI adoption obstacles on the enterprise buyers’ side: 1) need for clarity on return on investment, 2) model output quality control, and 3) data risk management.
According to Foundry’s August 2023 survey of 950+ global IT decision makers, a majority of IT decision makers agree that Gen AI-infused products create better business outcomes, but only 25% are piloting Gen AI tools. Amongst the companies piloting Gen AI tools, a majority focus on use cases that drive process efficiency and cost savings. That is because ROI is easily measurable. For example, streetwear brand Snipes leverages Gen AI to write product copy and do “two weeks’ worth of work in 2 hours,” according to its Chief Digital Officer Jenna Posner. Additionally, reinsurance company Munich Re uses LLMs to process unstructured text data and augment their underwriting processes, which saves manual review time by actuaries.
We often hear that the hesitation to adopt revenue-driving Gen AI tools is because executives feel a disconnect between their P&L and software usage. For years, SaaS companies have gotten away with usage-based pricing based on software outcomes (e.g., processing units used) that are not connected to operational outcomes. It is no longer sufficient for SaaS companies to bill on the basis of usage, but rather pursue performance-based pricing models that align with business outcomes of users. Take as an example, Intercom’s AI chatbot bills users on the basis of issue resolution -- meaning users are not billed until they feel satisfied that their query is fully resolved, regardless of how many processing units the query resolution required.
The burden of building more efficient solutions should be on software companies -- not on end users. A shift in pricing model from usage-based to outcome-based should help bridge the disconnect that executives feel for Gen AI and make implementation more imminent at enterprises.
To ensure Gen AI aligns with business results, executives should identify a set of operational metrics that directly drive P&L results. While an LLM-based assortment recommendation engine can be useful for an apparel brand, executives will unlikely be convinced of its ROI unless those recommendations specifically lead to more sell in and/or lead to new retail partnerships. Software should always be in service of the business outcomes, not the other way around.
We suggest three types of outcome-based pricing:
% of costs saved: companies are billed a percentage of costs saved using the tool.
Nuances like tiered pricing (e.g., $x if savings achieved from Gen AI copilot is below y%, $x+ if savings is at or above y%) can be introduced
Companies can also consider the human hours associated with internal jobs-to-be-done and evaluate how much of these jobs can be expedited by Gen AI solutions as a basis of pricing discussions
% of revenue: companies are billed a percentage of revenue generated using the tool
This method often relies on intermediate proxies (e.g., marketing-qualified leads generated via Gen AI copilot) or comparison against historical benchmarks (e.g., YoY increase in retailer sell in’s for a wholesale business thanks to Gen AI-enabled data insight collection)
Strategic milestones achieved: companies are billed when a significant milestone of strategic value is achieved (e.g., Gen AI visual campaign achieves x% clickthrough, y% of which from Gen Z consumers)
Ise AI builds technology that serves humans. To see how we build Gen AI tools to transform the retail industry, schedule a demo with us and follow the Ise AI Insights series to learn more.